The Diverse Impact of Artificial Intelligence Across Sectors
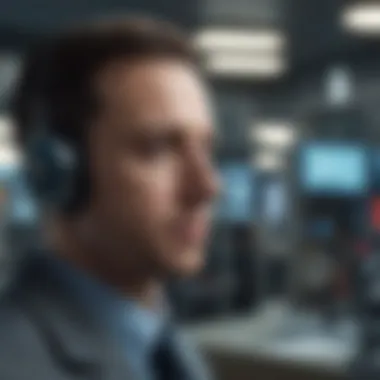
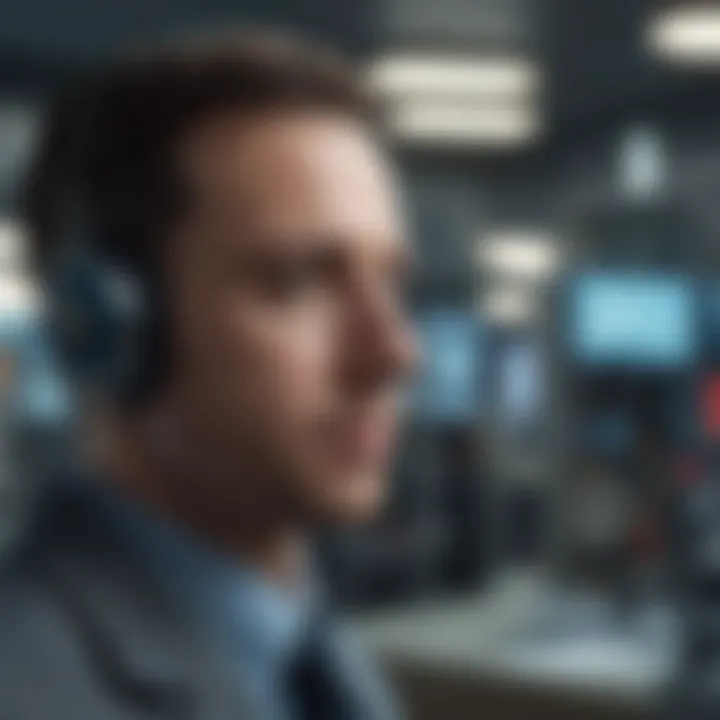
Intro
As technology continues to evolve, the integration of artificial intelligence (AI) into various industries has sparked a wave of innovation and transformation. This article embarks on a journey to explore the multidimensional applications of AI, diving into how this groundbreaking technology shapes sectors such as finance, healthcare, and education. With the intent to provide both a foundational understanding and insights into the challenges and ethical considerations that accompany AI, we’ll navigate through the historical context, the current landscape, and future trends that promise to redefine our interaction with technology.
The relevance of this topic is not merely academic; it resonates with anyone engaged in industries that are bound to be affected by AI advancements. As AI becomes increasingly woven into the fabric of everyday operations and decision-making processes, recognizing its implications becomes essential. This article aims to equip both novice and seasoned investors, as well as professionals, with knowledge that pertains directly to the transformative power of AI.
Investment Terminology
To understand the implications of AI, it's crucial to familiarize ourselves with certain terminology and concepts that often surface in discussions surrounding its applications.
Definition of Key Terms
- Artificial Intelligence: A branch of computer science that aims to create machines capable of performing tasks that typically require human intelligence, such as reasoning, learning, and problem-solving.
- Machine Learning: A subset of AI focused on enabling machines to learn from data and improve their performance over time without explicit programming.
- Deep Learning: A more advanced type of machine learning that involves neural networks with many layers. This technique is especially effective for complex tasks such as image and voice recognition.
- Natural Language Processing (NLP): A field of AI that helps computers understand, interpret, and respond to human language in a valuable way.
- Robotic Process Automation (RPA): The use of software robots to automate repetitive tasks usually performed by human workers.
Common Acronyms and Abbreviations
- AI - Artificial Intelligence
- ML - Machine Learning
- NLP - Natural Language Processing
- RPA - Robotic Process Automation
- IoT - Internet of Things
Familiarity with these terms is foundational for navigating discussions on AI implementations, particularly as these concepts continuously develop and find new applications.
Expert Advice and Strategies
In the world of AI, knowledge is power. Below are insights and strategies worth considering:
Tips for Novice Investors
- Start Small: Focus on understanding the basics of AI before delving into investments. Look for resources that simplify the jargon.
- Stay Current: Technology is ever-evolving. Follow reputable sources and experts to keep abreast of recent developments.
- Diversify Your Knowledge: Explore multiple sectors impacted by AI. Whether it’s healthcare, finance, or education, each offers unique insights.
Advanced Strategies for Seasoned Professionals
- Deep Dive into Data Analytics: Understanding how to analyze and interpret AI data can provide a competitive edge in making informed investment decisions.
- Leverage AI Trends: Identify which sectors within AI are gaining traction. Emerging fields such as AI ethics or autonomous technologies might present lucrative opportunities.
- Networking with Industry Leaders: Building relationships with professionals in the AI space can lead to insider insights and partnership opportunities.
As we continue throughout this article, each section will build upon these foundational concepts while exploring the significant impact of AI across various sectors.
The Fundamentals of Artificial Intelligence
Artificial intelligence (AI) stands as a cornerstone of modern technology, weaving its influence through numerous sectors, from finance to healthcare. Understanding the fundamentals of AI is not merely academic; it provides essential context to grasp its implications and applications in both everyday life and specialized fields. This section aims to strip back the complexities of AI, presenting its core elements and historical background while also preparing the groundwork for deeper exploration into its multi-faceted roles.
Definition and Scope
At its core, artificial intelligence refers to the capacity of a machine to mimic intelligent behavior. It encompasses a range of technologies designed to perform tasks typically requiring human intelligence, including problem-solving, learning, and pattern recognition. The scope of AI is broad, stretching across various domains such as robotics, data analysis, and natural language processing.
In terms of practical applications, AI drives innovations in sectors like healthcare, where diagnostic tools aid in identifying diseases quickly and accurately, and in finance, where algorithms analyze market trends to inform trading strategies. The dual nature—both a tool for productivity and a subject of ethical debates—highlights why understanding the scope of AI is crucial.
Historical Context
Early Developments in AI
The field of artificial intelligence began with dreams, aspirations, and experimental efforts. Early garnishments, such as Alan Turing's concept of a 'universal machine,' paved the way for the foundation of computational intelligence. Turing's work on the Turing Test initiated discussions about machine thinking and intelligence. In this respect, the charm of early developments lies in their philosophical directions; they aimed not just towards practical applicability but also questioned the nature of reasoning itself. This interplay of foundational logic and purely computational advancements made early developments a captivating choice for this article.
Milestones in AI Evolution
Several milestones punctuate the evolution of AI. From the 1956 Dartmouth Conference, which is often termed the birth of AI as a field, to the 1997 victory of IBM's Deep Blue over chess champion Garry Kasparov, each moment serves as a stepping stone towards the sophisticated AI systems we see today. A significant characteristic of these milestones is the continuous arousal and evolution of public interest, which has shifted perceptions about what AI can achieve. While these historical achievements brought marked advancements, they also unveiled new challenges, such as the public's needs to navigate and understand AI's impacting in their lives.
From Theoretical Concepts to Practical Tools
Transitioning from theory to practice was crucial for artificial intelligence to gain its current traction. Initially, many ideas surrounding AI were steeped in academic speculation; however, the development of practical tools, like expert systems in the late 20th century, showcased AI's potential. Such systems demonstrated concrete benefits in areas like medical diagnosis. The unique feature of this evolution was the pragmatic marriage of theoretical construct and real-world application, reinforcing AI's relevance in contemporary technology discussions. Nonetheless, this transition is not devoid of flaws, as the gap between expectations and reality often led to disillusionment when deploying AI solutions in various sectors.
Core Components of AI
Fundamentally, AI comprises various critical components that enhance its functionality and application across different fields.
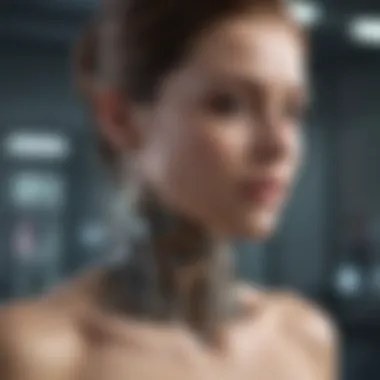
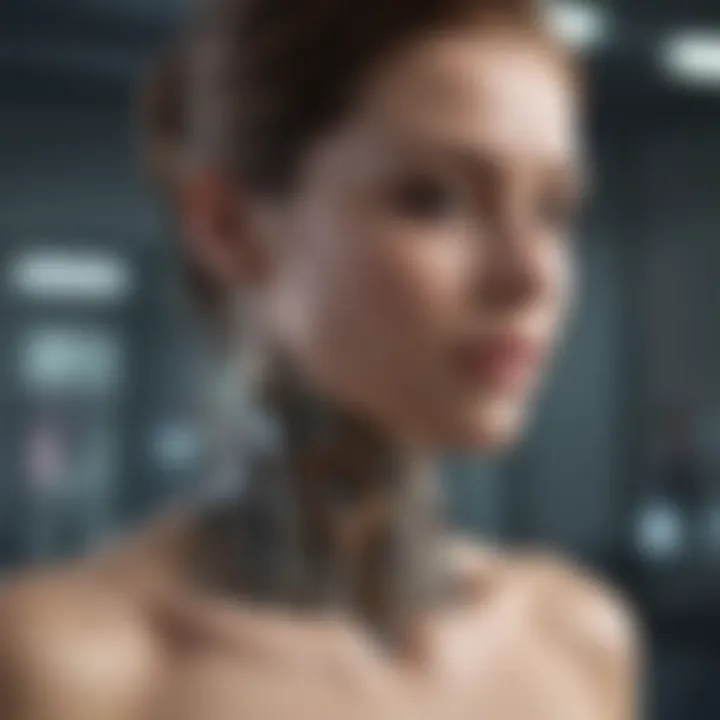
Machine Learning
Machine learning, a subset of AI, allows systems to learn from data and improve over time without explicit programming. By employing algorithms that adjust based on incoming information, this technology becomes a key player in predictive analytics. Many businesses choose machine learning for its ability to sift through massive datasets, making sense of them far faster than a human ever could. However, one significant downside is the potential for bias in data interpretation, which can skew results and impact decision-making.
Natural Language Processing
Natural language processing (NLP) fuels the interaction between computers and humans using language, making it easier to communicate. Its applications range from virtual assistants like Apple's Siri to advanced translation services. The powerful integration of human-like conversation capabilities puts NLP at the forefront of AI discussions. Despite its advantages, understanding the subtleties and complexities of human language can still pose challenges, which often leads to misunderstandings in communication with AI systems.
Computer Vision
Computer vision grants machines the ability to interpret visual information from the world, enabling applications in facial recognition, autonomous vehicles, and more. This technology's standout characteristic is its capacity to process and clarify countless images more effectively than a human brain can. However, the rapid pace of this technology also raises concerns about ethical implications, particularly relating to privacy and surveillance, reminding us of the need for responsible AI deployment.
"AI isn't just a technological advancement; it's a fundamental shift in how we interact with the world around us."
By laying out the foundations of what AI encompasses, this article sets the stage for a deeper examination of its practical applications in various sectors, illustrating how essential it is to understand both its capabilities and the challenges it presents.
AI in Financial Services
The financial sector is undergoing a radical transformation through the integration of artificial intelligence (AI). This technology is not just a passing trend; it is reshaping the very fabric of how financial institutions operate, enabling them to enhance efficiency and make data-driven decisions. The significance of AI in financial services can’t be overstated, as it offers numerous advantages such as accuracy, speed, and the capacity to analyze vast amounts of data. In recognizing these capabilities, it is crucial to delve into the specific applications of AI, such as algorithmic trading, risk management solutions, and fraud detection mechanisms. Each of these areas reflects the multifaceted role AI plays in enhancing financial operations and ultimately benefits consumers and institutions alike by creating a safer and more robust financial ecosystem.
Algorithmic Trading
Algorithmic trading refers to the use of algorithms to automate the trading process, executing large volumes of trades at speeds humans cannot match. The demand for executing trades in milliseconds has given algorithmic trading a foothold in the financial world. Traders and institutional investors can deploy complex mathematical models to make buy or sell decisions based on market conditions, historical data, and a host of other factors. Some core benefits of algorithmic trading include:
- Reduced Costs: By minimizing the number of human interventions, algorithmic trading tends to lower transaction costs.
- Elimination of Emotions: Algorithms operate on logic and data. Hence, they avoid the pitfalls of emotion-driven trading which can lead to poor decisions.
- Enhanced Performance: Algorithms can process vast datasets quickly, improving the potential for profitability based on real-time data analytics.
While algorithmic trading offers many advantages, it also brings about concerns regarding market volatility and the potential for technological failures.
Risk Management Solutions
AI's application in risk management revolves around identifying, analyzing, and minimizing risks faced by financial institutions. Financial markets are notoriously unpredictable, making robust risk management strategies crucial. AI technologies leverage machine learning algorithms and data analytics to assess risks associated with lending, investments, and market conditions. Key elements of AI in risk management include:
- Predictive Analysis: AI models can forecast risk scenarios based on historical data, enabling institutions to proactively manage potential threats.
- Real-Time Monitoring: Continuous monitoring of risk factors allows for immediate responses to emerging challenges, which is vital in dynamic markets.
- Customization: AI can tailor risk assessments to individual portfolios, ensuring that risk management strategies are relevant and effective for specific investments or clientele.
AI’s ability to adapt to changes in market conditions is invaluable for mitigating risks, which can save institutions from heavy financial losses.
Fraud Detection Mechanisms
Fraud is an ongoing threat in financial services, and AI plays a significant role in detecting and preventing fraudulent activities. Traditional systems struggle to keep up with the sophistication of fraudulent schemes, making AI-driven solutions more necessary than ever. Utilizing advanced algorithms and machine learning techniques, AI can analyze patterns and behaviors that indicate fraudulent activities. Here are some noteworthy advantages of AI in fraud detection:
- Advanced Pattern Recognition: AI systems can analyze transactions in real time, identifying anomalies that may suggest fraudulent behavior.
- Learning Capabilities: Machine learning algorithms adapt and improve over time by learning from new data, enhancing their ability to catch novel forms of fraud.
- Improved Accuracy: By significantly reducing false positives, AI enables financial institutions to focus their investigations on suspicious activities that truly warrant further scrutiny.
AI technology brings a proactive and effective approach to fraud detection, making it a vital component in maintaining the integrity and trustworthiness of financial services.
Healthcare Applications of AI
The realm of healthcare is experiencing a significant shift thanks to the integration of artificial intelligence. In an industry where the stakes are immeasurable and the margin for error is razor-thin, the deployment of AI tools fosters enhanced decision-making capabilities, decreased operational costs, and improved patient outcomes. As patients expect more personalized care amid growing demands, AI stands at the forefront, offering solutions that traditional methods simply cannot match. This section delves into three critical areas where AI is transforming healthcare: Diagnostic AI Tools, Personalized Medicine, and Healthcare Operations Optimization.
Diagnostic AI Tools
Diagnosing ailments accurately and swiftly can often mean the difference between life and death. Here, AI steps in with its diagnostic tools that can analyze vast amounts of data far faster than a human ever could.
AI algorithms are designed to examine medical imaging and pathology data with precision. For example, deep learning models are increasingly being used to interpret X-rays, MRIs, and CT scans. There's a certain exhilaration in observing how contemporary platforms like Google Health's AI technology are achieving remarkable accuracy in detecting conditions such as breast cancer significantly earlier than traditional methods. This isn't merely about data crunching—it's about potentially saving lives.
This increased efficiency doesn't just apply to imaging. AI also offers tools that can analyze genetic information, bolster the accuracy of diagnostic tests, and even identify disease outbreaks in real-time. By helping practitioners leverage data more effectively, AI is akin to an extra pair of eyes for doctors, enhancing their capabilities without overshadowing their judgment.
Personalized Medicine
The concept of personalized medicine redefines the notion of tailored healthcare. With AI, we are moving from a one-size-fits-all approach to a more nuanced methodology where treatments are customized based on individual characteristics, especially genetic profiles.
Imagine a patient with a particular type of cancer. AI models, utilizing large datasets, can predict how certain drugs will interact with the patient's unique biological makeup. These predictive models not only enhance the effectiveness of treatments but also minimize adverse effects—promoting engagement with patients who are looking for more control over their health prospects.
Moreover, AI can assist in pharmacogenomics, which studies how genes affect a person's response to drugs. This adds another layer of customization that can lead to superior outcomes and more efficient healthcare delivery. As we continue to gather more data and improve algorithms, the boundaries of personalized medicine expand, leading to a remarkable transformation in therapeutic practices.
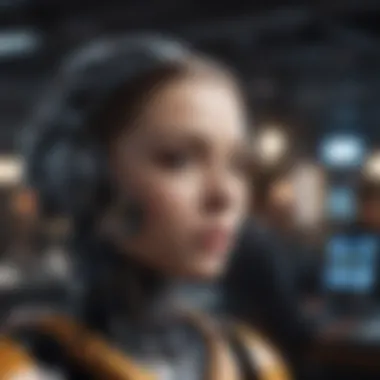
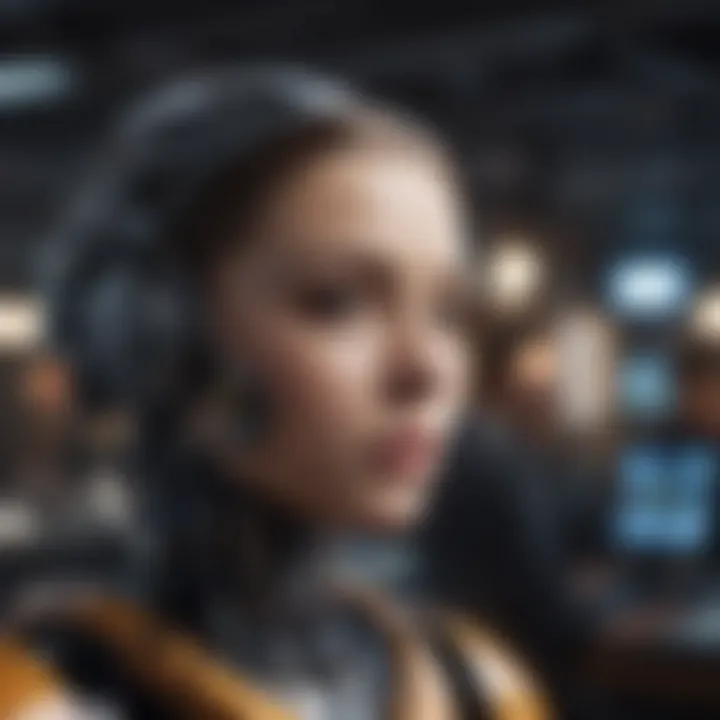
Healthcare Operations Optimization
The back end of healthcare operations often gets overshadowed, but it's just as vital to ensuring that patients receive high-quality care. AI can streamline operations, making processes smoother and reducing costs for healthcare providers. For instance, scheduling appointments using AI algorithms minimizes the hassle of double-bookings and ensures optimal utilization of medical staff.
Additionally, AI-driven predictive analytics can support resource allocation by forecasting patient influx and managing inventory in hospitals. This is particularly useful in emergency care services, where ensuring sufficient supplies can literally save lives.
Further, with the implementation of AI in administrative tasks, healthcare professionals can focus on what truly matters—their patients. Less time spent on mundane paperwork leads to better patient interactions and improved overall care quality.
"AI is pushing the limits of what is possible in healthcare. The quest for enhanced speed and accuracy is reshaping the landscape, making new heights attainable."
The Role of AI in Education
Artificial Intelligence has become an indispensable tool in the educational landscape, bringing forth various ways to enhance learning experiences and operational efficiencies. The integration of AI technologies in education has proven to be a game changer, driving improvements in how knowledge is disseminated, assessed, and customized to meet the diverse needs of learners. With the incorporation of data analytics and predictive modeling, AI is set to redefine traditional educational practices, ultimately tailoring them to foster more effective outcomes.
The importance of this role cannot be overstated. As educational institutions scramble to meet the varied demands of a globalized economy, AI offers tools that can augment teaching methodologies. For students, it translates to enriched and personalized learning journeys. For educators, it means relief from administrative burdens, allowing them to focus more on instruction and engagement.
Adaptive Learning Technologies
Adaptive learning technologies represent one of the most exciting applications of AI in education. These systems utilize algorithms to analyze the pace and performance of individual learners. By doing so, they create customized pathways that adjust in real-time, catering to students' unique strengths and weaknesses.
Some significant advantages of adaptive learning include:
- Personalization: Students can receive resources and assignments tailored to their current mastery level, which keeps them challenged yet motivated.
- Efficient Learning: By not spending excessive time on topics they have already mastered, learners can focus on areas in which they need the most improvement.
- Real-time Feedback: Instant insights into student performance enable both learners and teachers to identify knowledge gaps quickly.
These characteristics come together to foster an educational environment where learning is more efficient and aligned with each student's specific needs. It transforms the one-size-fits-all approach into a more nuanced system that recognizes diversity in learning styles and paces.
AI-driven Assessment Tools
Moving beyond the classroom experience, AI-driven assessment tools play a pivotal role in redefining how educators evaluate student learning. Traditional assessment methods often rely heavily on standardized tests that may not accurately reflect individual student capabilities or learning progress. AI-powered tools enhance this process by providing more comprehensive and nuanced evaluations.
Some critical benefits of these assessment tools include:
- Automated Grading: AI can handle grading of multiple-choice, fill-in-the-blank, and even essay-type questions, freeing educators from this time-consuming task.
- Detailed Analytics: Institutions can access rich data insights about student performance, highlighting trends over time and informing curriculum improvements.
- Fairness and Objectivity: AI can help reduce the bias that sometimes creeps into human grading, creating fairness in assessments.
In summary, the application of AI in education is not simply a trend; it’s a powerful evolution that holds the potential to reshape curricula, assessment methods, and the fundamental way education is perceived and delivered. By leveraging adaptive learning technologies and AI-driven assessment tools, educators can create a more targeted, efficient, and equitable learning experience for all students.
Ethical Implications of AI
The use of artificial intelligence is not just a technological advancement; it raises profound ethical questions that society must grapple with. Understanding these implications is essential, as they shape not only how AI is deployed but also how it affects different aspects of our lives. Ethical considerations foster accountability, which can ultimately influence public trust in AI technologies. This trust is vital for encouraging widespread adoption and ensuring that innovations serve humanity in positive ways.
Bias in AI Algorithms
Bias in AI algorithms is a critical issue that stems from the data used to train these systems. When datasets reflect existing societal biases, the algorithms learn these biases and replicate them in their outputs, which can lead to skewed results. For example, in hiring processes, an algorithm trained on historical hiring data may favor candidates from certain demographics while disadvantaging others. This can reinforce stereotypes and perpetuate inequality across various sectors, from employment to law enforcement. It’s crucial for developers to actively seek diverse datasets and implement fairness metrics to mitigate these biases.
AI and Privacy Concerns
Privacy is another significant ethical area surrounding AI. The integration of AI tools into everyday life often involves the collection of vast amounts of personal data. Consumers frequently willingly share information, yet many remain oblivious to how that data is used. For instance, AI-powered applications in healthcare may analyze sensitive medical records to enhance patient care, but if these systems are hacked or mishandled, sensitive information could be compromised. Striking a balance between benefiting from AI and protecting individual privacy rights is an ongoing challenge that requires legislation, transparency, and robust security measures.
Accountability in AI Usage
Accountability in the application of AI technologies remains murky. When an AI system makes a mistake—whether it involves an erroneous diagnosis from a medical AI tool or a faulty financial prediction—it’s often unclear who should bear the responsibility. Is it the developer, the company utilizing the AI, or the algorithm itself? As artificial intelligence systems become more autonomous, establishing a framework for accountability becomes paramount. Clear guidelines need to be in place to delineate responsibility and facilitate recourse when AI fails to deliver accurate outcomes.
"Without clear accountability, the potential for harm, whether intentional or accidental, could undermine the societal benefits of AI."
In summary, while AI promises to transform industries and enhance productivity, it also carries a weighty ethical burden. Addressing bias, safeguarding privacy, and establishing accountability mechanisms are crucial steps that need to be taken to ensure artificial intelligence serves as a force for good. Only by prioritizing these ethical implications can we navigate the complexities of AI development and deployment in ways that promote fairness, safety, and trust.
Future Trends in Artificial Intelligence
The dynamic landscape of artificial intelligence is continuously evolving, and understanding its future trends is imperative for anyone interested in leveraging its potential. The way AI is integrated into various sectors holds the promise of not just enhancing operational efficiency but also transforming entire industries. Key trends in AI will shape not only how businesses operate but also will significantly influence societal norms and values in the near future.
Advancements in Deep Learning
Deep learning, a subset of machine learning, has made remarkable strides in recent years. This technology mimics the way humans learn, allowing machines to process vast amounts of data. With improved algorithms and increased computational power, deep learning is becoming more effective in areas such as image and speech recognition, natural language processing, and autonomous systems.
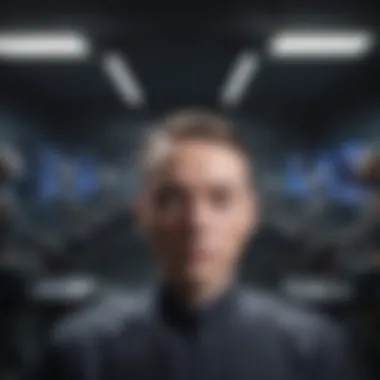
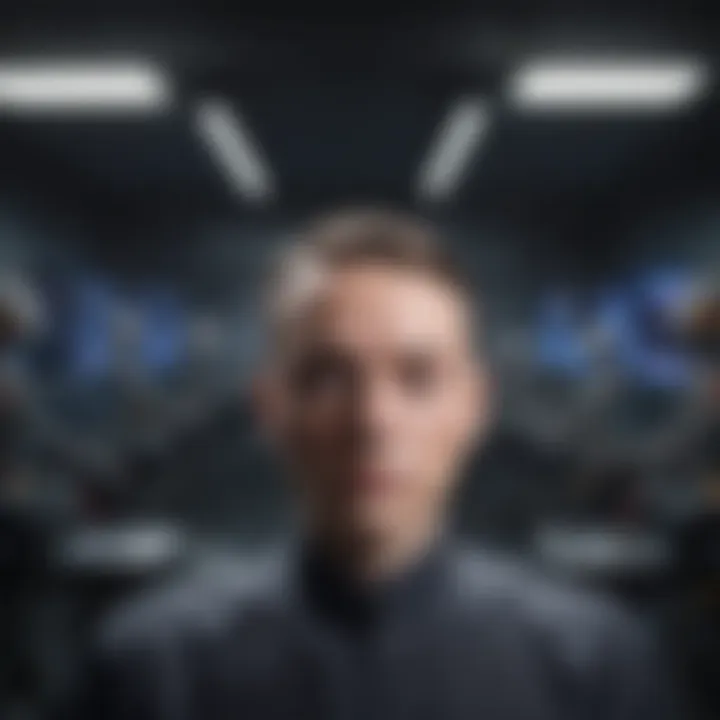
- Greater Accuracy: Enhanced neural networks have pushed the boundaries of what is possible, making voice assistants, like those used in smart devices, more conversational and capable of understanding context.
- Applications in Healthcare: In diagnostics, deep learning can analyze medical images with precision, which can aid doctors in identifying anomalies more accurately.
- Industry Influence: From marketing personalization to financial forecasting, industries are harnessing deep learning to develop smart solutions that cater to consumer needs.
"Deep learning models are becoming the backbone of AI advancements, paving the way for smarter, more responsive technologies."
AI in the Context of Big Data
As the volume of data generated continues to skyrocket, the interplay between AI and big data cannot be overstated. The sheer scale of data presents challenges and opportunities for organizations seeking to gain insights and drive innovation. AI plays a pivotal role in interpreting and processing this data, enabling more informed decision-making.
- Data Processing Efficiency: AI tools can sift through terabytes of information to extract patterns that humans might overlook. This feature is especially crucial in industries such as finance, where timely analysis can mean the difference between profit and loss.
- Real-time Analytics: Businesses can utilize AI-driven analytics to monitor trends in real-time, allowing for agile responses to market changes.
- Predictive Insights: With predictive modeling, organizations can forecast future trends and behaviors based on historical data, creating a competitive edge in their respective markets.
Potential for Autonomous Systems
The idea of completely autonomous systems, including vehicles and smart robots, is becoming a reality. The advancements in AI are paving the way for machines that not only assist but can operate independently in complex environments. This shift towards autonomy raises intriguing possibilities and considerations.
- Smart Transportation: Autonomous vehicles are being tested and integrated into logistics and ride-sharing platforms. This shift could revolutionize the way we think about transportation and urban planning.
- Manufacturing and Robotics: Functional robots are beginning to take on more sophisticated tasks on production lines, from assembling products to managing logistics.
- Societal Changes: As autonomous systems become commonplace, ethical considerations about job displacement and the responsibility of AI decisions will gain more attention.
Overall, the future trends in artificial intelligence are set to reshape our world dramatically. Both individuals and businesses will need to adapt to these changes, leveraging the understanding of deep learning, big data, and autonomous systems for success. By doing so, they can not only keep pace with evolving technologies but also positively influence their industries and communities.
AI's Impact on Employment
The rise of artificial intelligence has stirred quite a pot when it comes to its influence on the job market. As industries increasingly adopt AI technologies, the landscape of employment is changing dramatically. It’s not just a matter of machines taking over; there’s a complex interplay involving workers' skill sets, the very nature of jobs, and the economy. Understanding AI’s impact on employment is crucial for deciphering the future of work, especially in a world that increasingly relies on technology.
Job Displacement Concerns
One of the primary concerns that reverberates through discussions on AI and employment is job displacement. As AI systems become smarter, there's a considerable worry that they might take over tasks traditionally performed by humans. This is particularly evident in sectors like manufacturing, logistics, and even customer service.
- Automation of Routine Tasks: Many jobs that involve repetitive tasks are at risk. For instance, assembly line roles have been replaced by robots that can operate faster and more accurately.
- Shift in Skill Requirements: Notably, the demand for high-skill, low-manual labor jobs is increasing. Workers who may have previously filled low-skill roles need to adapt or risk being left behind.
- Economic Impact: There's a broader economic concern about the potential for increased unemployment, especially in lower-income communities where jobs are highly susceptible to automation. If a significant portion of the workforce finds themselves without jobs, the consequences would be felt across various layers of society.
The shadow that displacement casts can be daunting, but it’s equally important to take a step back and consider how the workforce can adapt and thrive amid these changes.
Emerging Job Roles in AI
Amid the anxiety surrounding job loss, there's also a silver lining: the emergence of new job roles that never existed before, thanks to AI. As technology advances, new avenues open up, shaping a future where human and machine collaboration becomes the norm.
- AI Ethics and Compliance Managers: With the increasing integration of AI into decision-making processes, there’s a crucial need for specialists who can ensure that these systems follow ethical guidelines and regulations.
- Data Curators and Annotators: AI systems require vast amounts of data to learn and function. This has led to roles focused on gathering, cleaning, and structuring data for AI algorithms.
- AI Maintenance and Oversight Roles: Technicians and engineers who maintain and repair AI systems will be vital as companies invest more in these technologies.
It’s essential to recognize that many of these roles require advanced education and training. While some jobs will vanish, new opportunities will flourish for those willing to adapt. This evolution in the labor market is not just about competition; it’s about collaboration between humans and AI.
"In the face of technological change, the focus should shift from fear of displacement to embracing the potential for creating innovative careers."
As we traverse through this technological transformation, it’s vital to equip the workforce with the skills necessary to thrive in an AI-driven landscape. Continuous learning and re-skilling will be paramount in navigating the challenges and taking advantage of the opportunities that AI presents.
Overall, the interplay between AI, employment, and the economy will not simply be a narrative of loss. There is potential for growth, creativity, and development on a scale that could redefine how we engage with work. The need for strategic foresight and adaptability in this landscape cannot be overstated.
Challenges in AI Implementation
The integration of artificial intelligence into various industries is not without its quibbles. As organizations rush to harness the power of AI, they encounter a number of hurdles that can prove to be quite challenging. Addressing these hurdles is crucial because it not only affects the efficiency and effectiveness of AI systems but also influences the broader acceptance of AI as a valuable asset. The challenges can be categorized into two main aspects: technical limitations and regulatory hurdles. Understanding these factors can significantly impact a company's success in implementing AI solutions.
Technical Limitations
At the heart of the matter lies the technical limitations of AI technologies. One of the most pressing concerns is the data quality and quantity required to train machine learning models. AI systems are notoriously hungry for data, and they thrive only when fed a rich and diverse dataset. If this data is biased or insufficient, the model's performance may suffer, leading to misjudgments in real-world applications.
Consider a hospital looking to adopt AI for diagnostic purposes. If the training data primarily comes from a specific demographic, the resulting model may not perform well for other patient groups. This can have dire consequences in healthcare, where diagnostics are a matter of life and death.
Furthermore, there’s the challenge of computational resources. Many AI algorithms, particularly deep learning models, require significant hardware capabilities. Investing in powerful servers can be a costly affair, particularly for smaller organizations that operate on tighter budgets. This aspect can create a stark divide, where only well-funded companies can fully leverage AI's potential.
Another technical challenge is the interpretability of AI models. Especially in sectors like finance and healthcare, stakeholders need to understand how AI systems arrive at their decisions. The so-called "black box" nature of certain AI technologies can breed mistrust. If stakeholders can't grasp how decisions are made, they may be reluctant to use these systems, fearing potential pitfalls.
Regulatory Hurdles
Regulatory issues present another serious challenge in the path of AI implementation. The field of AI is evolving faster than most legal frameworks can keep up with, leading to a significant gap between innovation and regulation. Compliance with existing laws can be complicated, especially in sectors like finance and healthcare where privacy is paramount.
For instance, consider the General Data Protection Regulation (GDPR) enacted by the European Union. Organizations using AI must ensure that their data collection and processing practices comply with these stringent regulations. Failing to do so can result in hefty fines or legal battles, creating a daunting prospect for businesses looking to implement AI systems.
Moreover, the ethical considerations surrounding AI usage are also under scrutiny. Questions about accountability in AI decisions and bias in algorithms are at the forefront. If a financial institution uses an AI model that inadvertently discriminates against a specific group, the repercussions can be severe, including legal actions and a tarnished public image.
"Successful AI implementation hinges on a clear understanding of both the technological capabilities and the regulatory environment that guides its use."
Addressing these challenges head-on helps pave the way for better, responsible use of artificial intelligence in vital sectors.